Unpacking AI Training Biases in Midjourney
You all may know that I’ve been a bit obsessed with Midjourney in recent months. After all, it is kinda amazing: We in live in a magical age, in which you can give a computer a set of simple, plain language, text instructions, and it will regurgitate, well, whatever you want– in this case, images. One of the things I keep running into, though, is bizarre biases in the AI training of the model. This is the kind of thing that is going to have to factor into questions around equity and ethics in artificial intelligence– and probably regulation that encourages the development of those ethical parameters– and it all begins with my attempt to get the AI model to render an image of a Detroit neighborhood. You can already see where this is going, I’m sure!
If you’re not familiar with Midjourney, click here for some other stuff I’ve written about the platform.
OpenAI Lawsuit: A Dubious Argument On A Monumentally Complex Question
Limitations of the Platform
In spite of beaucoup ethical questions about implications of AI for the interweb itself, which I could write a whole book about (I’m sure someone’s already using ChatGPT to do just this!), AI models today are neither Skynet, nor are they wizards. They are perhaps best likened to extremely sophisticated calculators. The models are all “trained,” which basically means that the developers (and armies of poorly paid trainers who review output) have to look at every image or text output generated by the model and say, “yeah, that looks about right for a rendering of a donut with pink icing” for an image, or, “wow, that answer is bizarre and super messed up and not accurate at all! Benito Mussolini did not invent Takis!”
Generally, this training works, and we’ve seen massive improvements in many areas, whether we’re talking about ChatGPT reducing its incidence of “hallucination,” or from Midjourney Version 4 to 5 in the past several months alone. As I’ve noted in the past, Midjourney in particular struggles with the discrete spatial boundaries of things like sidewalks, roads, and other infrastructure. A good example of this is how the AI renders cars parked on the sidewalk or grass. While this is common in Detroit neighborhoods and in downtown Detroit, where the US Marshalls gleefully thumb their noses at law enforcement and wheelchair users by parking on the sidewalk in front of the federal courthouse, it’s obviously not where cars are meant to park. My article on Midjourney imagining cities also referred to content generated by ChatGPT specifically, as I asked the LLM to postulate why many cars were omitted from some of the images. It had a fascinating response.
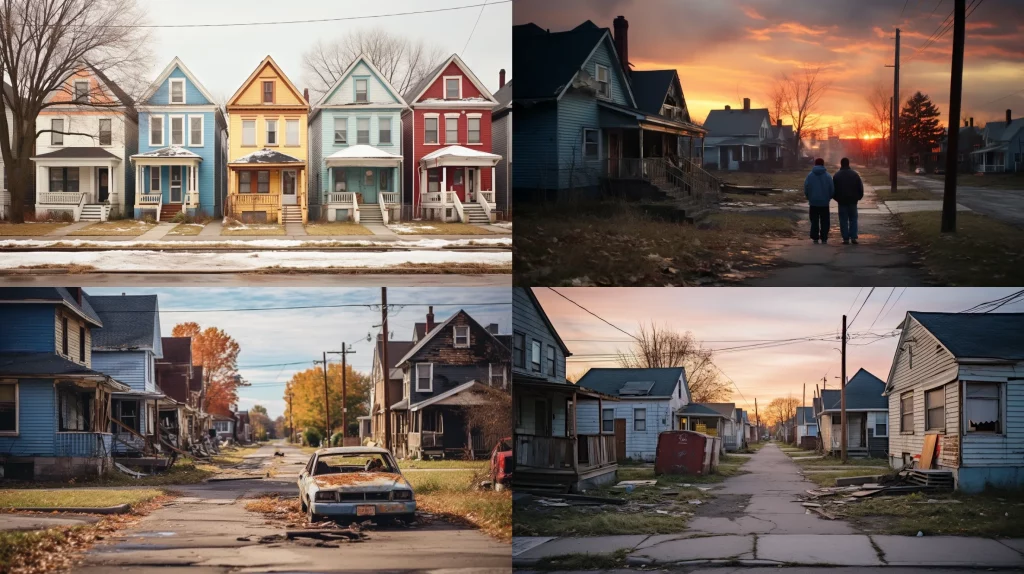
Detroit
Ever the curious urban planner who can’t keep himself from sticking his nose into The Discourse, I was curious to know what the robot thinks of the city I call home. The problem, of course, was that out of 21 prompts asking Midjourney to /imagine prompt: professional photograph of a Detroit neighborhood –ar 16:9, I only got one lone image that wasn’t of a bombed out hellscape of an urban environment. That’s 1 out of 84, or, a rate of 1.19% “positive” images over negative (you will remember that Midjourney renders four images for each prompt, in what I call a “quad”
Now, you might be thinking: Well, of course 83 of the 84 images are bombed out hellscapes! That’s the stereotype of Detroit, right?!
It surely is the stereotype— but it’s not even remotely accurate as a matter of geographic probability, to say nothing of architectural accuracy. Even the New York Times, with its coastal chauvinism, has conceded on multiple occasions that there’s a lot of cool stuff in Detroit! Put differently, this would mean that there are fewer than 2 square miles of Detroit that aren’t bombed out hellscapes, and about 136 square miles that are. While there are certainly, I’d venture to guess, dozens of square miles of the city that fit the stereotype that Midge has imagined for us here, I’d say that the majority of residential neighborhoods in the city that still have houses in them do not fit this stereotype image.
The stereotype, however, reflects the training. And that’s a big problem as AI systems become increasingly powerful and popular.
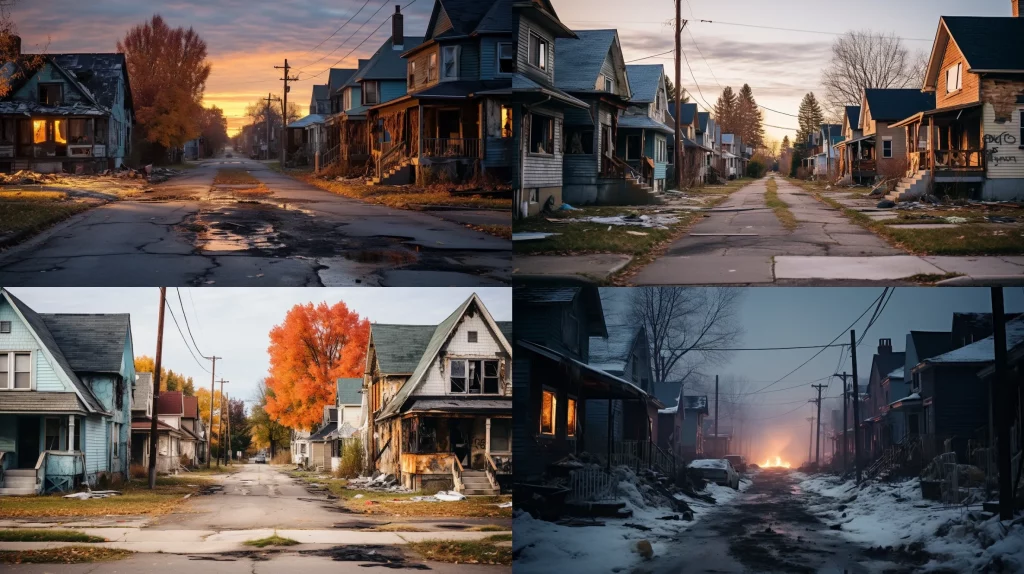
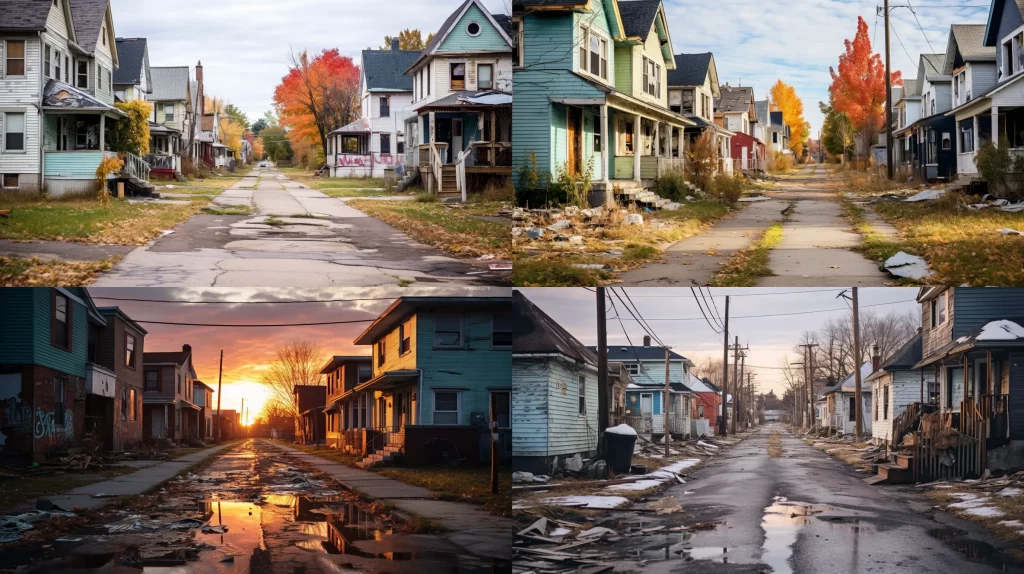
How do other cities fare?
Better than Detroit, certainly, and that’s no accident as far as the training is concerned. It seems that most prompts that include “photo” and “neighborhood” inevitably gravitate toward this “generic view down an unnaturally narrow street, with some working-class configuration of midcentury-or-later houses in modest states of repair or disrepair.” I am thinking of this as the Midjourney equivalent to how so much television in the early 90s was shot in British Columbia and then they just pretended it was California or New York or wherever with minor tweaks. (Oh, Agent Mulder, you’re in Los Angeles, you say, but it’s a misty rain and you’re surrounded by evergreen trees and verdant parkland? I don’t know about that).
It’s annoying and I can’t explain why– except for perhaps a lack of training.
For other cities, though, let’s first check out Windsor, a.k.a. South Detroit, hometown of a nameless city boy who took the midnight train going anywhere.
Windsor
Windsor, Ontario did quite well in my test. A few of the images produced were the generic format mentioned above. But the two images below— produced in the first two of only four prompts- could easily have been taken in Walkerville. The shot on the right looks almost like Wyandotte Street except it’s a bit too wide. I only did two prompts for Windsor and got several believable images plus a few generic ones. That easily beats the 1.19% “positive” rate in Detroit.
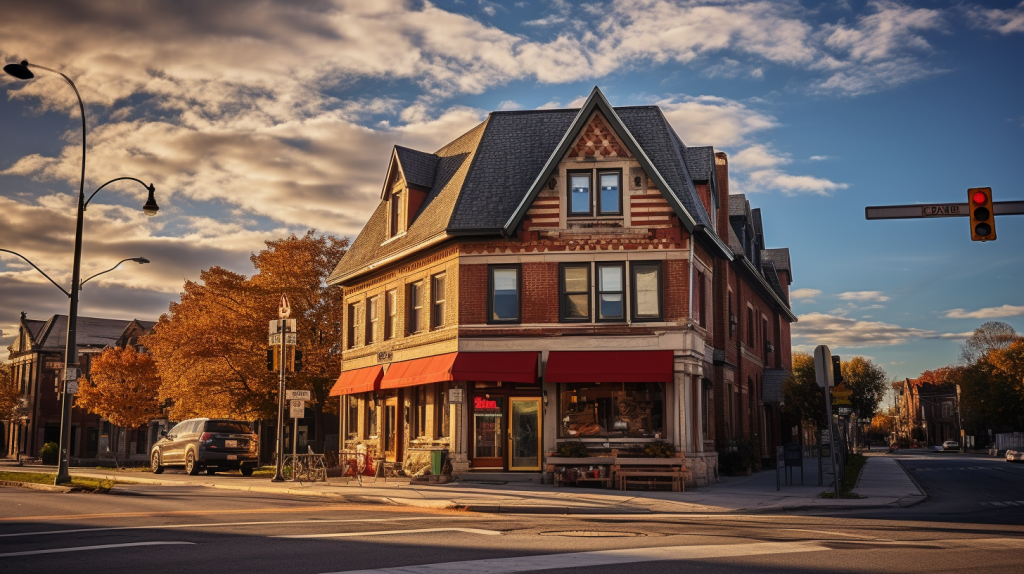
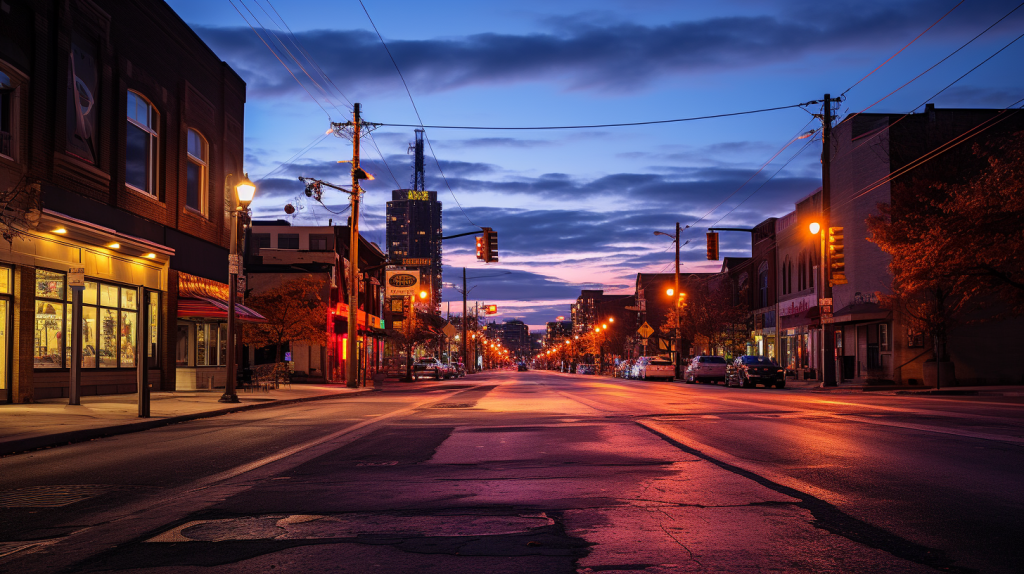
Compared to my Detroit review, the images of Windsor might even make James Howard Kunstler, the Howard Stern of urbanism, rethink his trash talk. Again, though, to someone who has spent a lot of time in Windsor, these look, well, about accurate!
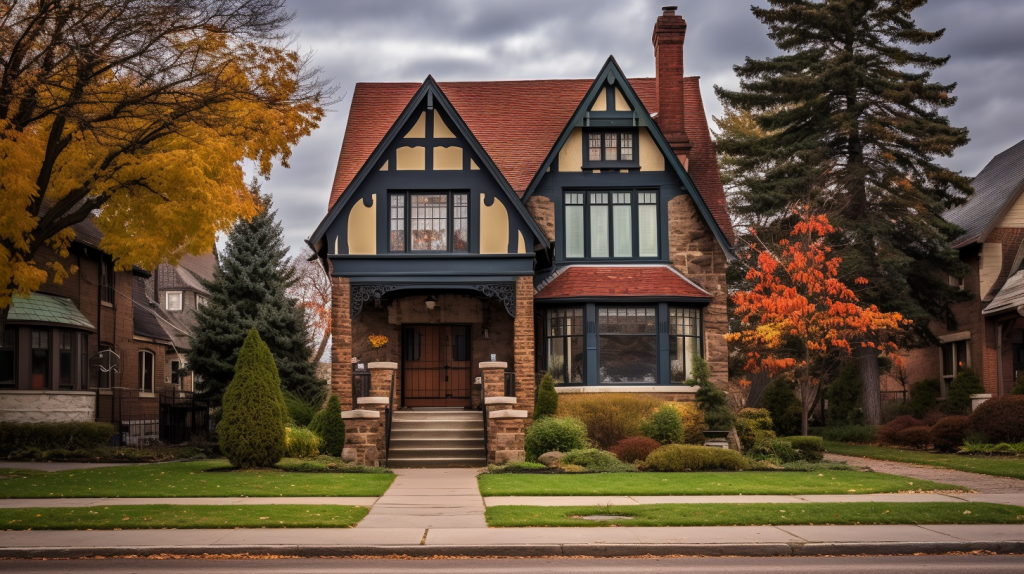
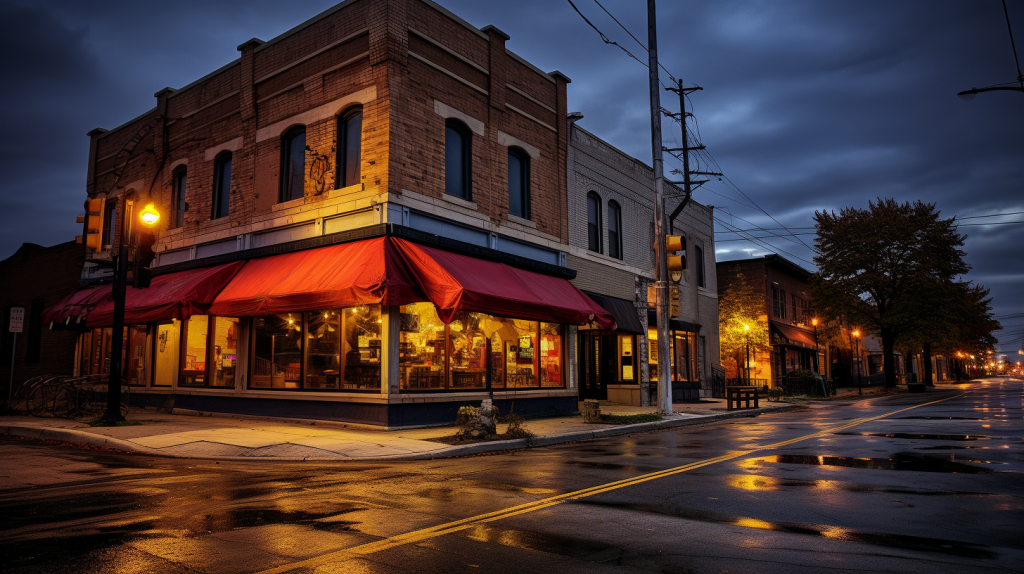
Chicago
In spite of the fact that right wingers love to beat on Chicago and claim that it’s some sort of literal hell on earth, there are a few million people who live there and probably take a lot of fairly accurate pictures of it, making it look like, well, not a horrible place. The big differences between the Chicago images and any of the other cities I tried were that the Chicago images often seemed to involve some serious Big City imagery in the form of high-density buildings or background shots of downtown. I only rendered four prompts for Chicago and I got some surprisingly believable results. Chicago has probably the strongest grid of any American city, including wide arterial streets, densely populated neighborhoods, strong tree cover, and, of course, often a distant skyline of downtown and the lakefront.
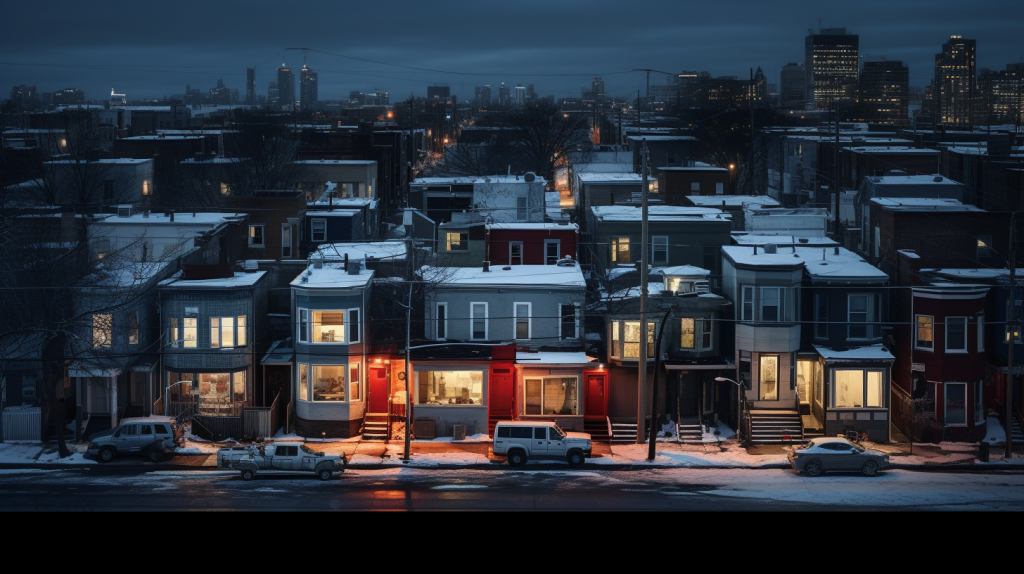
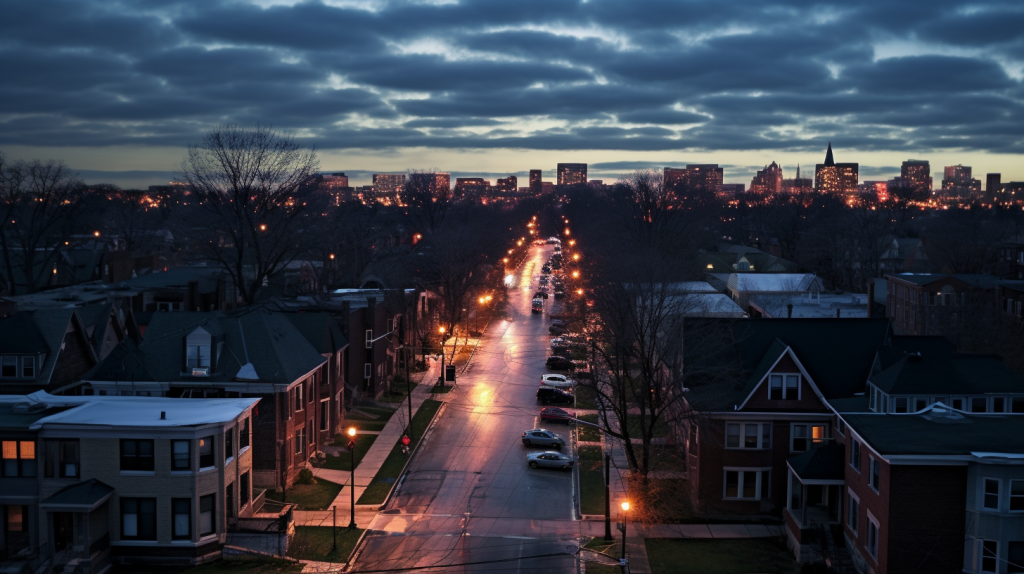
Okay, so, the Chicago winter is no joke, so I’m gonna allow that Midjourney’s best three images for the city were all in winter. I did three prompts for Chicago and got a few “generic working-class neighborhood” (we’ll just say “GWCN”) images, but also got two “this is definitely meant to be the hood” images, even though they didn’t particularly look like what the hood looks like in Chicago. Just generic lower-income disinvested neighborhood images, which are definitively a step down from the GWCN mentioned above. Again, blowing away the 1.19% positive rate I got for Detroit.
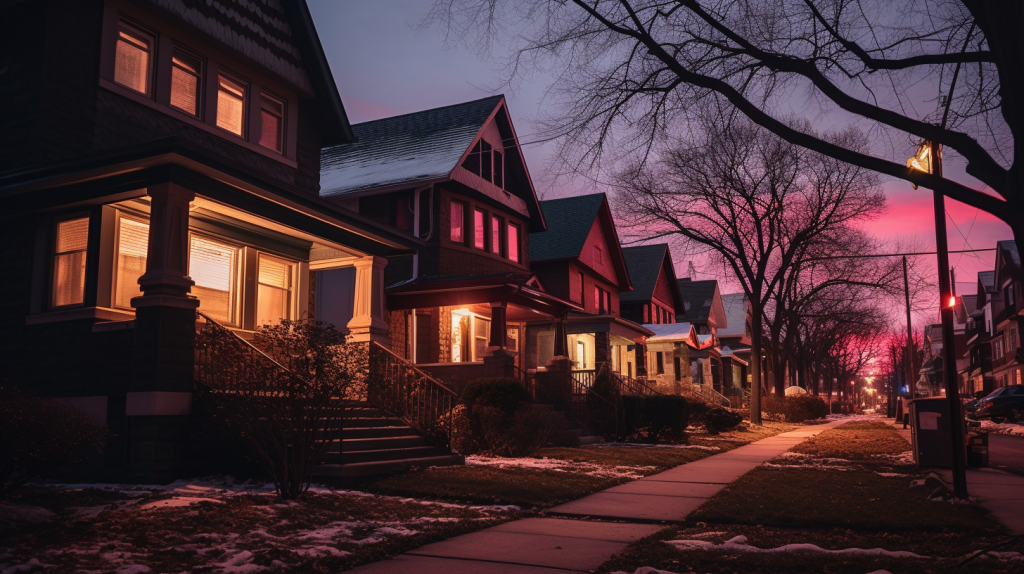
St. Louis
St. Louis, a city I will always love the same way I would love a spouse with severe substance abuse problems, didn’t do great, but it still fared way better than Detroit. Most surprising to me was the fact that the St. Louis images eschewed anything resembling what I think of as the Mound City– namely, the stately, solid brick buildings that can be found in either the wealthiest or most destitute neighborhoods.
Interestingly, I had more luck when I threw specific terminologies into the prompt (Left: “Tower Grove South” or right: “Central West End”). Surprisingly to me as someone who has spent hours photographing the historic neighborhoods of the city, I came up with nothing in particular when I tried to get images of the characteristic brick buildings mentioned above, nor when I tried to go for specific areas that I knew had a very characteristic style, for example, Lafayette Park, which is surrounded by palatial Second Empire homes. When I went for the prompt, “historic brick houses in North St. Louis,” I did get renderings of pretty houses. They didn’t look entirely familiar to me, but they didn’t look horribly wrong, either. It was a step in the right direction.
Returning to the Motor City to try this out? I tried this same trick for Detroit and got nowhere, whether I was trying “Woodbridge” or “West Village,” both of which have very characteristic architectural typologies. Midjourney did not play ball.
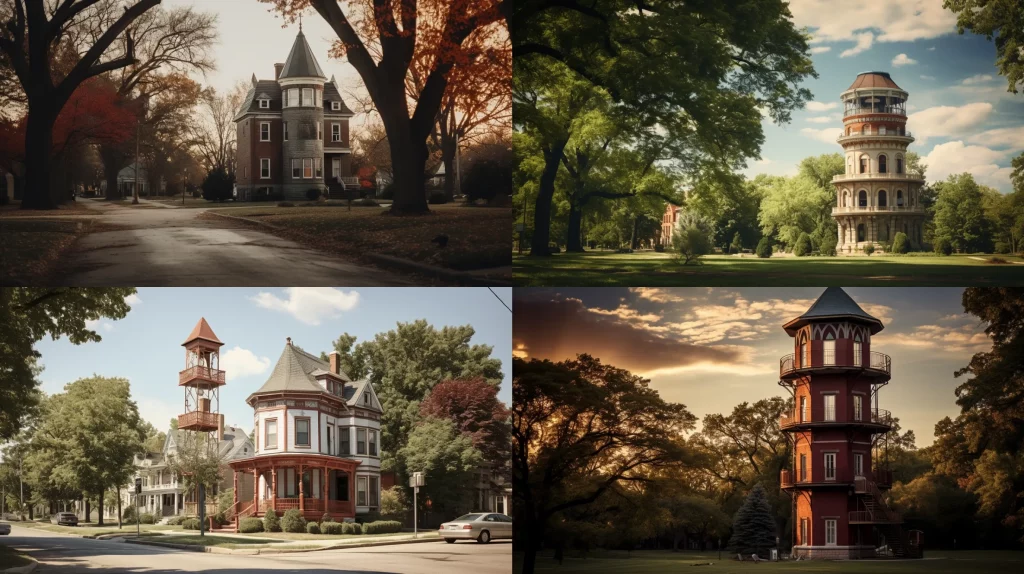
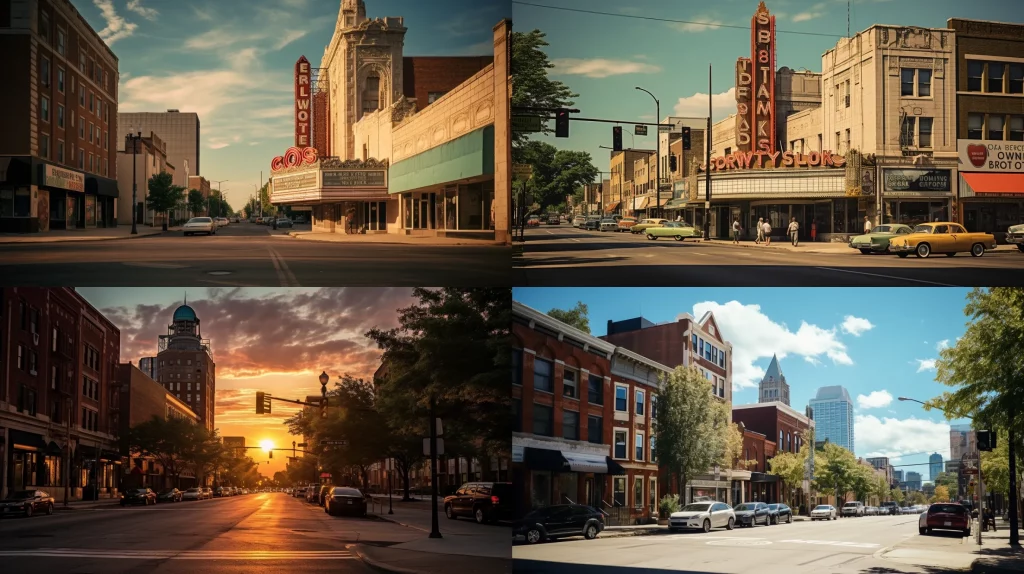
Cleveland
Taking Midjourney down to West Sixth Street! Cleveland’s images were a struggle to move beyond the GWCN toward which Midjourney seems to gravitate. I was able to get something a bit more believable when I tried, for example, “Ohio City neighborhood.” Ohio City is a fancier part of Cleveland that will eschew most of the GWCN results. Interestingly and probably by complete accident of whatever stochastic and probabilistic processes are gyrating within the Midjourney brain, the image on the left features at least one house that looks a little bit in the blighted category.
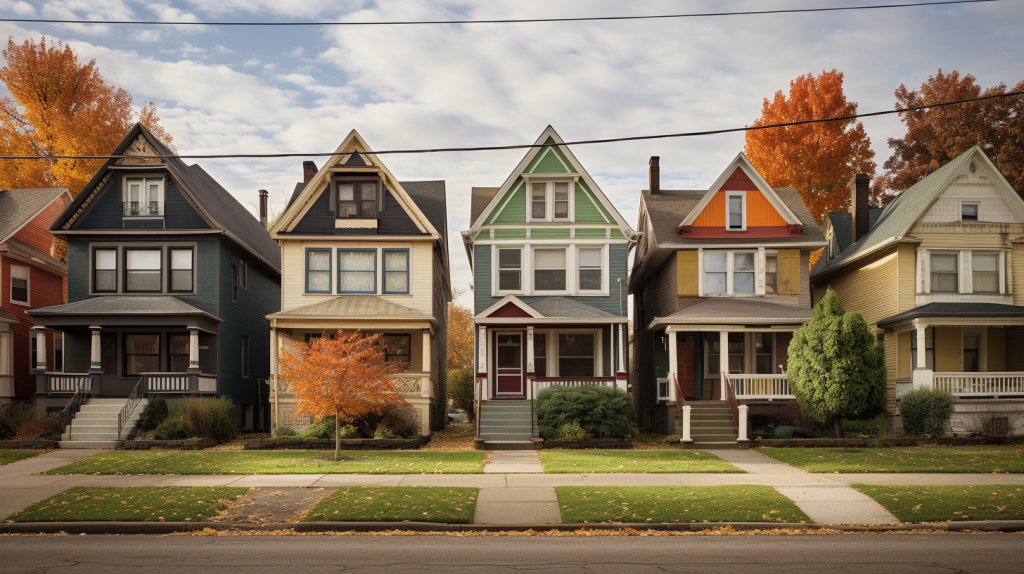
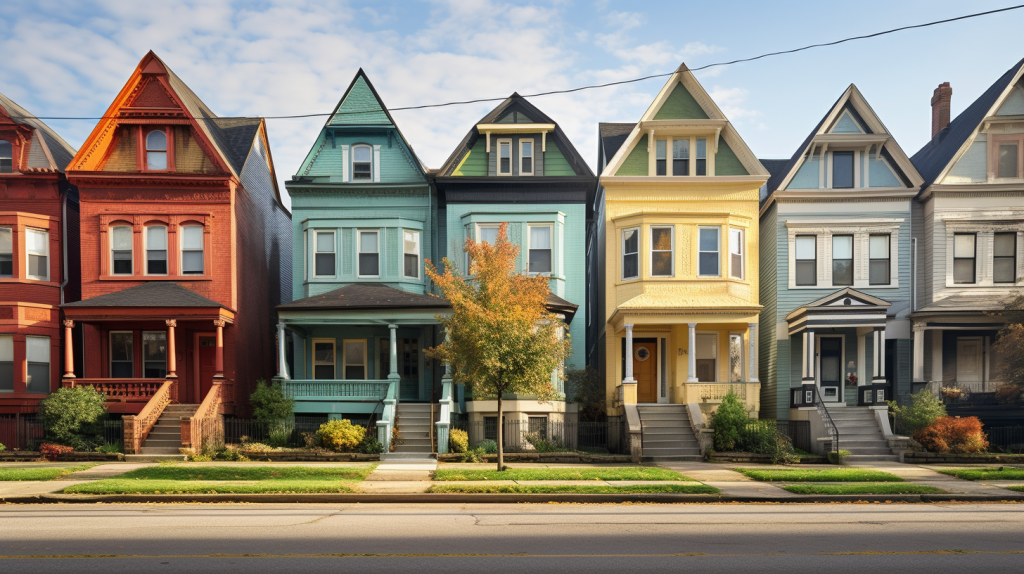
Minneapolis
Still struggling with the GWCN results, I tried “Uptown Minneapolis” and got surprisingly decent results in all of my attempts. Is this a matter of uptown being a historically fancier neighborhood than, say, Minneapolis in general? Or, again, was the training just bad for the general term “Minneapolis”?
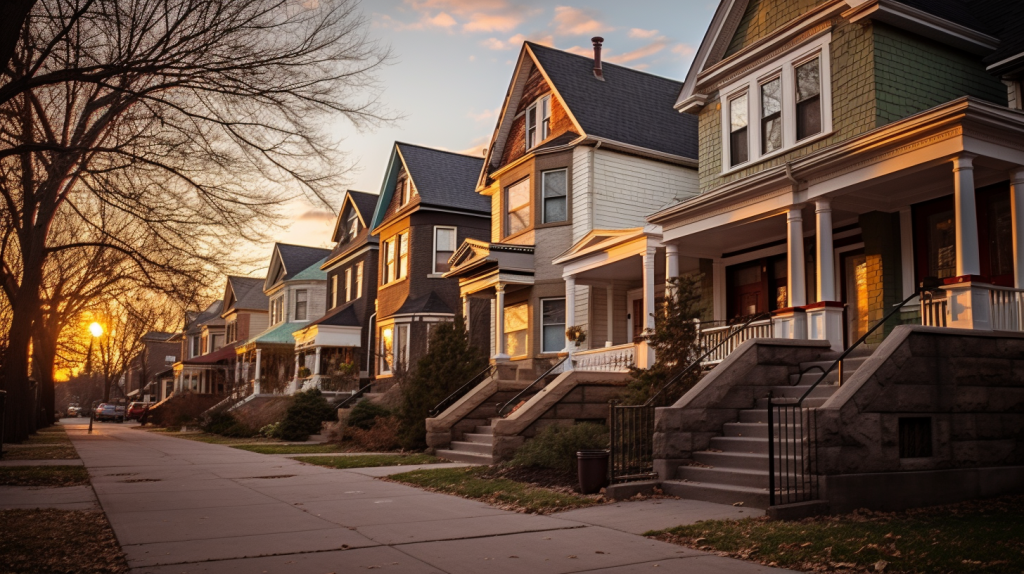
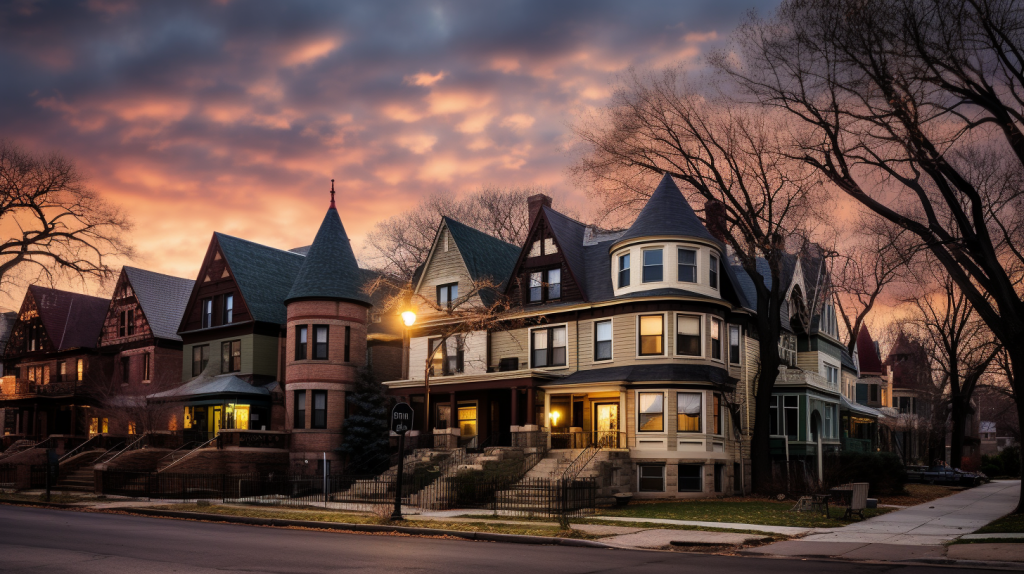
More likely than not, there was no conscious attempt on the part of the trainers to integrate bad, inaccurate, racist, or whatever other variety of bigoted ideas into the AI training. It’s possible that no one checked on this while developing the model. And I suppose it’s also possible that this was just a matter of suburbanites who weren’t thinking about what cities actually are. If you live your whole life in the ‘burbs and watch Fox News, for example, you might think that all cities look like this:
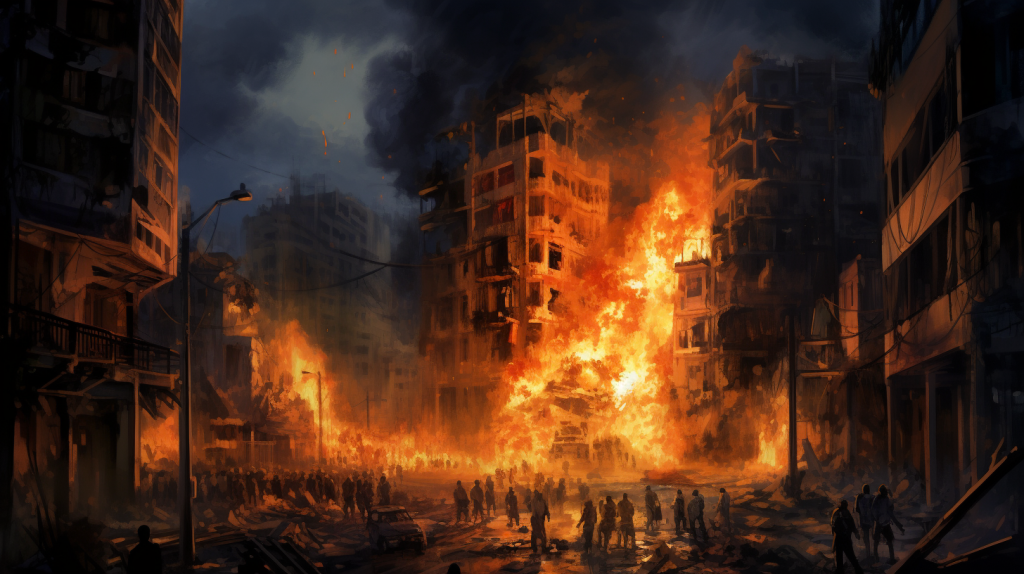
Conclusion: Better Training Means Better Outcomes And Less Bigotry and Stereotyping
Of course, none of the companies developing these products are at present terribly forthright about how they do their training. You can even ask ChatGPT about its training, and it doesn’t tell you much. This should be a key objective for anyone pushing for regulation of AI products, but it’s also the right thing to do: to explain how your model works, and to enlist expertise of a diversity of humans in thinking about how to get the most accurate responses out of the AI models.
For example, scientists should (and probably do) review output from scientific product being explained by something like ChatGPT. But for Midjourney, I feel like they should employ someone to review images of cities (among other things) that go into training– and make sure they actually reflect the architectural typologies, flora, topography, and whatever other elements, of the cities they’re supposedly representing. As we talk about the value of equity in an ethical society, this should extend to representation in how we portray the built environment, whether it’s a matter of humans talking about cities, or robots “imagining” them.